By Dr. Paul Monaghan, Fish Welfare Initiative Research & Development Lead
January 2025 update: Please see this more recent post for an update on the findings of this earlier study.
Summary
We previously conducted a proof-of-concept study to assess whether data predicted from satellite imagery could enable us to detect water quality issues remotely. The results of that study are now in: We found a strong correlation between the satellite-predicted and the empirical data, suggesting that satellite imagery could be a key part of increasing the scalability and cost effectiveness of our farm program moving forwards.
This is a blogpost discussing the study’s findings. The report of the study may be viewed here.
FWI is investing more in R&D this year. We will be running several studies throughout the course of the year in order to inform improvements to our programming, and will keep our blog updated with our progress.
Background
Fish Welfare Initiative’s core program, the Alliance for Responsible Aquaculture (ARA), focuses on improving water quality in fish farms. Our ground teams collect water quality data and provide farmers with corrective actions when needed. Currently, the ARA supports 135 fish farms in the Kolleru and Nellore regions of Andhra Pradesh.
In the current model, FWI data collectors visit each fish farm approximately once a month. This approach limits our scalability, as increasing the number of farms requires more data collectors. Additionally, the once-a-month visits mean that water quality issues between visits may go unnoticed, potentially exposing fish to poor conditions.
To address these challenges, we explored the possibility of using satellite imagery to remotely monitor water quality. If successful, this method could allow us to collect data more frequently without additional human resources, making the ARA more scalable and impactful.
Driven by our commitment to evidence-based programming, we initiated a study in February to test this approach. We hypothesized that if satellite-predicted water quality data were accurate and reliable, we could incorporate remote satellite monitoring as a cost-effective scaling mechanism in the ARA.
We are excited to share the key findings from this study.
The Study
To determine whether satellite imagery could accurately and reliably inform water quality decisions, FWI collaborated with Captain Fresh, an Indian seafood company experienced in using satellite imagery in the aquaculture industry. We conducted a proof-of-concept study involving 20 fish farms, all part of the ARA in the Kolleru region of Andhra Pradesh.
The study focused on six key water quality parameters: ammonia, dissolved oxygen (DO), chlorophyll-a (Chl-a), phycocyanin (PC), pH, and temperature. Data for these parameters were collected both empirically (through direct measurements) and remotely (via satellite imagery analysis provided by Captain Fresh). Data collection occurred every five days, aligning with the Sentinel-2 satellite's flyover schedule, resulting in five rounds of data collection for each farm.
For each parameter, the collected data were divided randomly into two groups. One group was used to train a predictive model, while the other was used to validate the model. We assessed the models using four statistical measurements: correlation coefficient (r), coefficient of determination (R²), root mean squared error (RMSE), and mean absolute percentage error (MAPE). Ideal models have r values close to ±1, R² values close to 1, and low RMSE and MAPE values.
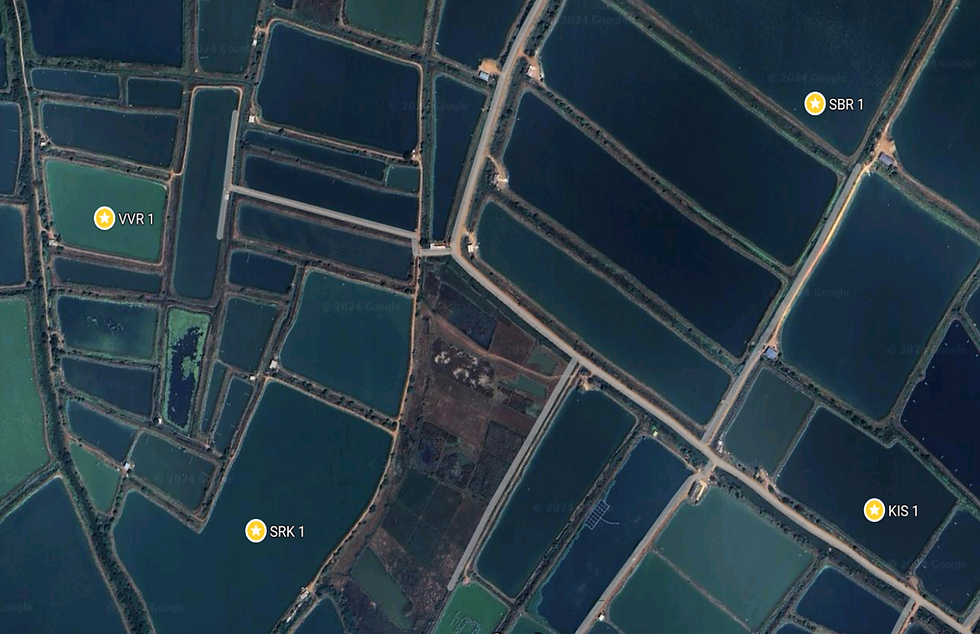
Our Findings
For four of the six water quality parameters—ammonia, DO, Chl-a, and PC—predicted and empirical data were sufficiently correlated to suggest that remote monitoring has utility. Most encouragingly, the predicted and empirical data for both PC and Chl-a showed high correlations, with r values of 0.99 and 0.96, respectively, and R² values of 0.98 and 0.92, respectively. The data for DO and ammonia were also strongly correlated, with r values of 0.90 and 0.92, and R² values of 0.81 and 0.85, respectively. These results indicate that remote monitoring of these parameters is feasible.
For two of the six water quality parameters—pH and temperature—there was no correlation between predicted and empirical data. The r values for these parameters were 0.22 and 0.41, and the R² values were 0.05 and 0.17, indicating significant deviation of predicted values from actual values. This suggests that remote monitoring of pH and temperature using these models is not currently viable.
The four water quality parameters with high r and R² values—ammonia, DO, Chl-a, and PC—also had low RMSE and MAPE values. This implies that the models' predictions were close to the actual values, supporting the potential utility of remote monitoring for these parameters. In contrast, the high RMSE and MAPE values for pH and temperature further indicate that the models for these parameters are not accurate.
Overall, the findings from this proof-of-concept study are highly encouraging, indicating real potential to utilize remote monitoring for ammonia, DO, Chl-a, and/or PC via analysis of satellite imagery as part of FWI’s flagship ARA program.
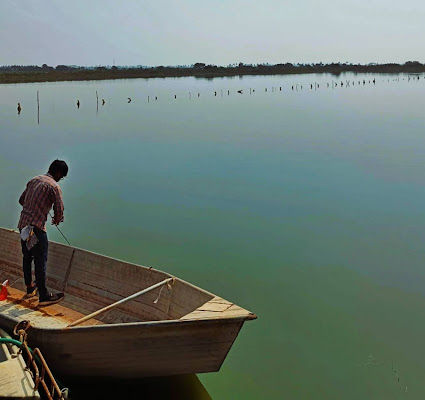
Next Steps
We believe that this concept will have utility for the ARA, but it’s a little too early to roll it out in the ARA just yet. Before incorporating remote monitoring of fish farms into the ARA, additional work is needed to improve the models for predicting these water quality parameters to ensure accuracy and reliability. Specifically, the models need further training with larger data sets and data collected over a longer period to avoid any bias from the short study window (three weeks).
Additionally, we need to understand how weather, particularly cloud cover, may impact the accuracy of satellite-predicted water quality values. Cloud cover is a known limitation of using satellite imagery for remote data collection, and it did impact data collection during this study. Regardless of model accuracy, understanding the significance of this limitation is crucial before making major changes to the ARA program.
Although not quite ready for programmatic purposes just yet, the findings from our proof-of-concept study allow us to move forward with greater confidence, guided by evidence. We’re excited by the potential of incorporating this technology into our programming to help us scale our work and improve the welfare of many more fishes.
We are now discussing internally how we plan to proceed with this intervention. As these plans develop, we will keep our blog updated with our progress.
Want to read the full study report? See here.
Comments